When considering what to cover in my first blog post, I kept coming back to topics related to spread trading. As a former full-time trader who made a living formulating and executing spread strategies, and as the person who leads the product management function for proprietary trading at TT, which includes automated trading, I think a lot about themes related to spreading.
TT’s Autospreader is one of the most widely used spread tools largely because it delivers sophisticated functionality and extreme performance in a very user-friendly package. I decided to write about dynamic hedging because I believe it’s an important concept for spread traders to understand and apply. Furthermore, I believe the way Autospreader addresses dynamic hedging with the Hedge Rule Builder tool is pretty powerful.
In this post, I’ll walk through some examples to illustrate how Hedge Rule Builder can be used to apply Pre Hedge and Post Hedge rules. But before I start on that topic, I’ll back up a bit for the benefit of readers who haven’t yet taken a deep dive into spread trading and hedging.
Spread Trading, Autospreader® and Hedge Rule Builder
The basic function of any spread trading tool, including TT’s Autospreader, is to execute orders to buy and sell a synthetic instrument composed of at least two exchange-listed securities, or “legs”. A spreader actively quotes at least one leg. When it accumulates inventory, it “hedges” by sending orders in the other leg in an attempt to buy or sell the synthetic instrument at the desired price.
Basically, a spreader performs two functions: it assumes risk, then reduces it as the spread order gets closer to completion. It provides liquidity, then seeks liquidity.
When trading a spread, hedging entails sending orders in legs as a reaction to an execution in an actively quoted leg. This is liquidity-seeking behavior, and therefore has a cost, which is mostly the price of crossing the bid-ask spread. While the main point of hedging is to reduce risk, a close second is to minimize the costs of reducing that risk.
How can we try to reduce our costs of seeking liquidity? One possibility is to vary our aggressiveness based on market conditions.
Autospreader’s Hedge Rule Builder offers two powerful ways to customize hedging behavior and aggressiveness: the Pre Hedge Rule and the Post Hedge Rule. The Pre Hedge Rule is evaluated after a quote fill but before any hedge orders are sent; the Post Hedge Rule is evaluated after hedge orders have been sent and acknowledged by the exchange. In other words, the Post Hedge Rule is only evaluated when there are “resting” hedge orders. Used together, these rules give us the opportunity to reduce liquidity costs, boosting bottom-line results.
Let’s walk through a few specific liquidity-seeking techniques to illustrate the concept.
Pre Hedge Rule
We’ll use the soybean crush for our examples. This is a common strategy where a position in soybean futures is offset by equivalent positions in soybean meal and soybean oil. We’ll define our spread as follows (using the soon-to-be-released X_TRADER® 7.17), with Hedge Round enabled to round hedging quantities rather than truncate them:
When we get a small fill on our quoting leg, we may decide to be less aggressive (where “aggressive” is defined as being less willing to cross the market) in pricing our hedge orders and making one-lots and two-lots “work for better” by applying a negative payup tick. Note that in this context, HedgeWorkingQty is the quantity Autospreader is about to submit:
Even if our hedge need is relatively large, we can be less aggressive if the opposite side bid/offer is strong:
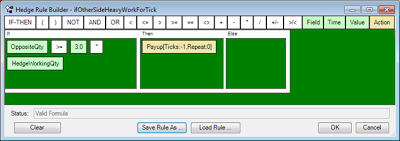
In other cases, by detecting that the market has moved away from us, we can be more aggressive. Note that at the time of the Pre Hedge Rule’s evaluation, HedgePrc is the price Autospreader will submit before including payup ticks:
Post Hedge Rule
After hedge orders have been sent, Autospreader can monitor market conditions and react based on user-defined logic. This allows us to continue to adjust our aggressiveness in seeking liquidity based on new information. Remember that when configuring a Post Hedge Rule, we need to provide a “repeat value” if we want the behavior to be dynamic—that is, performed more than once as conditions change.
One way to keep a hedge order “competitive” is to track its price against the best price in the market. It’s easy to write a rule such that if a hedge order “falls out” of the first five price levels, we get more aggressive by attempting to cross an assumed one-tick-wide market at the new level:
We can also base our behavior on time. We can improve our price one level once a second for the first minute of being “hung”:
Conclusion
As someone who still dabbles in trading, I really appreciate the fact that the Pre Hedge and Post Hedge rules allow the user to tweak Autospreader functionality. These logic “hooks” give the trader the needed flexibility to maximize opportunity and minimize costs.
These techniques are just a few examples of what is possible, and they can even be combined into more complex behaviors.
If you’d like to explore some of the more sophisticated applications of Pre Hedge and Post Hedge rules, feel free to submit a comment on this blog post. Or, you can consult the Online Help module on our website.
Tags: Algos & Spread Trading
 |
Tulane Algorithmic Trading Club members Willow Zhang (1st
Place) and Yuki Yang (2nd Place) used X_TRADER® to out-
perform the competition in the club’s first official trading contest.
|
Tulane University is one of Trading Technologies’ most active University Program partners. At Tulane, our X_TRADER® software is used in classroom instruction, and it’s installed at the A.B. Freeman Trading Center laboratory. The lab is accessible to all students, including members of the Tulane Algorithmic Trading Club (TATC).
The club supports research, facilitates discussions and encourages hands-on development of automated trading strategies. As a student organization, membership is open to all interested Tulane students.
TATC members gain real-world skills by writing and developing trading algorithms while learning from collaborations with their peers and faculty advisors. The students are challenged to trade in simulation mode against live market data using industry-standard technologies, including X_TRADER and TT’s application programming interfaces (APIs). The algorithms that they develop are deployed in simulation against real-market data, and the strategies are ranked in terms of profit to identify the students who’ve best met the challenge.
Recently, the club held its first official trading competition. Entry was open to all Tulane students. Participants were encouraged to be creative in their use of information and technology when they developed their automated strategies, which ranged from high-frequency styles that monitored market liquidity and took advantage of arbitrage opportunities to those that were a bit more long-term in nature and driven by technical indicators.
And the Winner Is…
After the contest concluded, TATC President Zachary Poche and Secretary Geoffrey Lewis reported: “…the Tulane Algorithmic Trading Club held its first trading competition. Algorithms were pitted against one another in a battle of mathematical wits and technical savvy. The competitors met in the trading laboratory at 12:00 PM, and, after a quick fine tuning of Trading Technologies by our resident tech wizard Kevin Ammentorp, trading began promptly at 12:15. After 45 minutes of trading, the best algorithm was evident and club member Willow walked away with the top prize, our respect and adoration, with an overall profit of $55,000. The first runner up was Yuki with a profit of $8,000.”
Willow’s winning strategy utilized the Average Directional Index (ADX) and the Commodity Channel Index (CCI), while Yuki’s runner-up strategy was a Moving Average (MA) indicator built entirely in ADL™, TT’s visual programming platform.
The students who participated in the contest said they appreciated the opportunity to apply what they learned in class using real-world tools in a real-world setting—to get real-world results.
Of particular interest to me is how enthusiastically the students employed ADL. It enabled them to design, test and deploy their trading algorithms in C# without writing a single line of code. Geoff and Zack told me that one of the biggest advantages to using ADL was the speed at which the students were able to generate, test and deploy their strategies once they were defined. These students haven’t graduated yet, but they know that in the marketplace, “speed to market” is vital, and they leveraged ADL to obtain a speed advantage.
Clubs like Tulane’s Algorithmic Trading Club highlight the collaboration of university, students and business. As a voluntary endeavor, the students display an entrepreneurial spirit with faculty guiding that spirit. I’d like to think companies like ours, that provide the technology and training, give them the tools to empower that spirit and bring their ideas to life. That’s a winning strategy for everyone.
ACKNOWLEDGEMENT: The Tulane Algorithmic Trading Club is steered by Zachary Poche (President), Joshua Aiken (Vice President) and Geoffrey Lewis (secretary). Professors Joe LeBlanc and Geoffrey Parker are the faculty advisors.
Tags: Algos & Spread Trading, Trade Execution, TT CampusConnect
“Nobody can catch all the fluctuations.”
– Jesse Livermore
TT partners with universities around the world through our University Program. We provide our software, free of charge, to dozens of schools to help them prepare students for careers in the global derivatives industry.
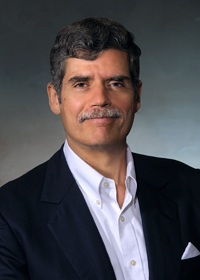 |
Professor Robert Webb |
Our collaboration with the University of Virginia has been particularly beneficial. Professor Robert Webb has utilized our software in classes at both Virginia’s McIntire School of Commerce and the Darden Graduate School of Business. Students get experience in electronic trading as well as an understanding of automated trading through TT’s software.
In this guest post, Professor Webb and Alexander Webb share their thoughts on the recent benefits and challenges that high-frequency trading brings to today’s markets.
It has been said that today’s high-speed financial markets can change in the blink of an eye. That is wrong. A blink of an eye is too slow. In a market increasingly dominated by high-frequency trading (HFT), prices can change sharply in a millisecond, but it takes between 100 and 200 milliseconds for a human eye to blink.
Simply stated, you are literally missing trading opportunities in the blink of an eye.
With speed like this, can humans even hope to make money in a market dominated by high-frequency traders? Yes—but it entails trading smarter.
The Growth of High-Frequency Trading
HFT is growing because it is immensely profitable. A 2012 study by Baron, Brogaard and Kirilenko reports that HFT firms made $29 million in profits from the e-mini S&P 500 stock index futures market during August 2010 alone.1 And they did so bearing little risk. The average Sharpe ratio was a phenomenal 9.2. To be sure, the HFT firms earned a small amount per contract traded—on average $1.11—but given that they traded thousands of contracts each day, the small profit per contract grew quickly.2
HFT firms are not all the same. They vary from firms that are passive liquidity-providers to firms that are aggressive liquidity-takers. There are HFT firms that target human traders and firms that target other high-frequency traders. The Baron, Brogaard and Kirilenko study reports that the most aggressive HFT firms—which were largely liquidity takers—made the most money.
Milliseconds matter in the fast-paced world of HFT. This has led many HFT firms to co-locate their computer servers near the exchange servers in order to reduce exchange latency, i.e., the time it takes for an exchange to report information to participants.
How much is a millisecond worth? A 2012 study by Frino, Mollica and Webb found the introduction of co-location in the futures markets on the Australian Securities Exchange (ASX) in February 2012 resulted in a two-millisecond advantage to the HFT firms co-locating their servers near the ASX computer.3 Given that HFT firms are paying a minimum of A$10,000 per month for this privilege (and usually more), they must believe it is money well spent.
But the rise of HFT isn’t the only negative news for humans. The study suggests that the introduction of co-location on the ASX futures markets has increased liquidity with no apparent adverse effect on volatility. This increased liquidity would result in an annual savings of A$12 million in the cost of trading the four principal ASX financial futures contracts. These are estimates for the societal savings from the increased liquidity that HFT firms provide.
Trading Obsolescence
The reality is that while HFT may result in increased liquidity, it also presents many obstacles to the human trader. Strategies that were profitable before HFT are now obsolete.
Among those strategies with questionable profitability today are:
- Arbitrage: Markets move so quickly that the opportunity to arbitrage between them is difficult if not impossible for those who do not utilize HFT.
- Market making: HFT imposes excessive risks on those traders who provide two-sided markets in that the market maker cannot react to a change in the order flow as quickly as the high-frequency trader.
- Getting the “edge” in the market (i.e., buying on the bid or selling at the offer): With the exception of illiquid markets where the bid/ask spread is wide, the only situation where a trader can participate on the bid or the offer is when that market is turning.
- Event trading: Competing against HFT in terms of speed of response to scheduled economic reports and conventional news is impossible since HFT systems can process the information and react to it quicker.
Human traders need to trade strategically to avoid the dangers HFT presents.
Trading Opportunities
Is there hope for human traders? HFT systems may be fast, but they don’t always get it right. Sometimes, it seems that the market does not always know which direction it ultimately should move. Take, for example, the reaction of EuroFX futures to the monthly employment situation report on February 3, 2012. The euro initially rose in reaction to the January 2012 employment report and then fell. The same was true of gold. Moreover, this was not a one-off event, but similar examples occurred at other times. The key takeaway is that you may have more time than you think to react.
Humans are likely to be best at reacting to freak situations and unexpected market shocks. Not all algorithmic traders are high-frequency, but all high-frequency traders use algorithms. When the winds of change hit the market, humans are still more adaptable, flexible and able to change with the times. While algorithms can be reprogrammed, they can’t be reprogrammed fast enough to take advantage of a contemporaneous shock.
Algorithms are often unable to discern real news from fake news. For example, a tweet from a fake Muddy Waters Twitter account led to a 25 percent selloff in shares of Audience Inc. Reuters quoted Hammerstone Group founder, Jamie Lissette, as saying, “It’s good to some degree, because it makes people realize that we can’t just have a computer doing something like that just based on ‘Muddy’ and a symbol.”4
Trading Smarter
What are the weaknesses of high-frequency traders? Basically, many of them have gone after the easy money—market making—although with an eye toward minimizing losses. They are constrained by their algorithms. You need to think differently.
You need to get your hands dirty by observing the market’s reaction to various news events and spotting oddities. Other things equal, you are probably better off with a reason or hypothesis why prices should behave in a certain fashion than not. Your hypothesis also needs to be tested. It has never been easier for non-programmers to test relationships or develop simple trading systems. For instance, Trading Technologies offers ADL™, a
visual programming platform that allows non-programmers to easily create algorithms by dragging building blocks onto a design canvas and connecting them to create executable strategies. The blocks convert to pre-tested code, and the desired features are all pre-programmed for you. Software like this is one way to develop and test potential trading strategies.
 |
TT’s ADL™ visual programming platform allows traders and programmers alike to
develop, test and
deploy automated trading programs without writing a single line of code.
|
QIM co-founder and “hedge fund wizard” Jaffray Woodriff, in his interview with Jack Schwager in Hedge Fund Wizards, argued that traders should “look where others don’t.”5 This is excellent advice. Equally important is the need to test potential ideas in a rigorous fashion in order to avoid introducing biases into the analysis.
Some HFT algorithms attempt to identify human orders from other HFT orders. Trading smarter also means not succumbing to some of the decision pitfalls to which humans are prone, like submitting orders for an even number of contracts or trading when volume is lower during the day (i.e., outside the open and close), etc. Easley, Lopez de Prado and O’Hara [2012] suggest some ways that human traders can avoid being victimized by HFT.6
Perhaps the most important thing to remember is that you get to choose when to trade, and you should only trade when you have an advantage. Whether you’re trading intraday or long-term, you should only pull the trigger when you are confident the odds are in your favor.
About the Authors
Robert I. Webb is a Paul Tudor Jones II research professor at the University of Virginia. His industry experience includes positions at the World Bank, the Chicago Mercantile Exchange, the Office of Management and Budget and the CFTC. He earned his PhD in finance from the University of Chicago. He edits The Journal of Futures Markets and has authored numerous books including his most recent book on high-frequency trading he co-authored with his son Alexander,
Shock Markets: Trading Lessons for Volatile Times.
Alexander Webb is a writer. His interests include finance, emerging markets, politics, business, technology and international travel. Living in Asia for half a decade, Alex earned a baccalaureate degree in international business and global management from The University of Hong Kong. He has studied at the Chinese University of Hong Kong, Beijing Language and Culture University and at Shanghai Jiao Tong University. He also studied in Japan at Ritsumeikan Asia Pacific University.1 Baron, Brogaard and Kirilenko, “
The Trading Profits of High Frequency Traders,” Working Paper, University of Washington, August 2012.
2 Baron, Brogaard and Kirilenko [2012] report that the small profit per contract “equates to $46,039 per day for each HFT in the August 2010 E-mini S&P 500 contract alone.”
3 Frino, Mollica and R.I. Webb, “The Impact of Co-Location of Securities Exchanges’ and Traders’ Computer Servers on Market Liquidity,” Working Paper, University of Sydney, November 2012.
4 Reuters, “A Tweet from Someone Posing as Short Seller Carson Block Sent a Stock Tumbling 25% Today,”
BusinessInsider.com, January 30, 2013.
5 Schwager, J.,
Hedge Fund Wizards, New Jersey: Hoboken, John Wiley & Sons, 2012.
6 Easley, Lopez de Prado and O’Hara, “
The Volume Clock: Insights into the High Frequency Paradigm,” March 30, 2012. The Journal of Portfolio Management, Fall 2012, Johnson School Research Paper Series No. 9-2012.
Tags: Algos & Spread Trading, TT CampusConnect
As further indication that Chicago is becoming increasingly recognized as a legitimate hub for tech talent in the U.S., the GOTO international software conference
came to Chicago for the first time this week. The conference included speakers from many top names in the industry discussing the latest advancements in the software development community. Trading Technologies was proud to be a sponsor of the inaugural GOTO Chicago conference, and I was thrilled to have the opportunity to give a talk on
visual programming languages (VPLs), and how TT has managed to utilize this technology in the algorithmic trading community with our
ADL™ (Algo Design Lab).
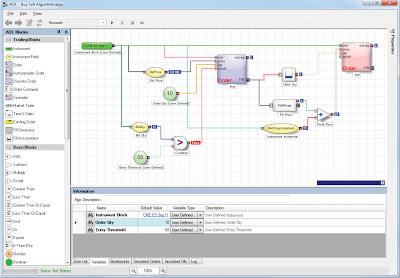 |
Using visual programming, TT’s ADL allows traders and programmers alike to rapidly design, test and deploy automated trading programs without writing a single line of code. |
Applying visual programming to professional industries has proven difficult; the fundamental tenets of VPLs (approachability and ease-of-use) tend to belie the goal of providing a usable platform for getting “real work” done. As educational tools for children or first-time programmers, VPLs often shine, but applying them to very specific domains (like low-latency trade development) often ends in frustration and something that looks more like spaghetti than anything else.
As I discussed in my GOTO talk, the way in which ADL has overcome these challenges—and a good model to follow when applying a VPL to any domain—is by adhering to three important characteristics:
- Domain specificity
The fundamental building blocks in ADL are modeled with the automated trader in mind, utilizing concepts and vocabulary that come naturally to participants in this industry, without exposing complexity that is unnecessary to the business needs. If not domain specific, general-purpose VPLs tend to be cumbersome when trying to build something of business value.
- Hiding the “dirty stuff”
As most any algorithmic trading developer will admit, managing tasks like “in-flight orders” and exchange-specific nuances add complexity to any automated strategy. What makes matters worse is that these complexities don’t offer the opportunity to differentiate your strategy from others. In other words, there is no value to be gained by solving these problems, so why spend time worrying about them? ADL aims to hide these “dirty” details to let you focus on what matters most: designing your trade quickly and safely.
- Mitigating the “Deutsch Limit”
Any development framework is only as good as its ability to provide unlimited flexibility without sacrificing maintainability; the larger and more complex your strategy becomes, the more you rely on your development environment to help you navigate your code. This should be no different for a visual development environment, and ADL strives to give you a rich set of tools that helps you manage even the most complex algos. With the proper tools in place, the Deutsch Limit is no longer a factor when it comes to managing visual complexity.
I wrapped up my talk at GOTO Chicago by explaining that I never intended to build a visual programming language. However, after realizing our industry lacked the tools which could provide a safe, robust environment for developing low-latency trading strategies, and which could empower every trader—regardless of technical know-how—to compete in the world of automated trading, ADL was the inevitable result.
A special thanks to the GOTO team for putting together such a fantastic few days of programming discourse.
Tags: Algos & Spread Trading